About Us
At Eprecisio, we are experts in MLOps, committed to helping businesses operationalize AI seamlessly. Our team specializes in leveraging cutting-edge tools like Kubeflow, MLflow, Azure MLOps, and AWS-based MLOps solutions to create scalable, robust, and automated pipelines for machine learning.
With certifications in GPU workload optimization, infrastructure management, and cloud-native architectures, we bridge the gap between data science and production.
99.9%
Uptime Guarantee
40%
Average Cost Reduction
24/7
Support Available
100+
Successful Deployments
Our Services
MLOps Pipeline Automation
Design and implement CI/CD pipelines specifically for machine learning workflows. Expertise in Kubeflow Pipelines and MLflow tracking for reproducibility and scalability.
Model Deployment & Management
Streamline model deployment with real-time monitoring and continuous integration. Support for hybrid setups, from Azure MLOps to AWS SageMaker.
Infrastructure Management
GPU-optimized workloads for high-performance AI training and inference. Manage on-premise data centers with GPU clusters, hybrid cloud, and public cloud environments.
Monitoring & Optimization
Real-time drift detection, error analysis, and predictive insights for your deployed models. Ensure peak performance and reliability of your AI systems.
Data Engineering for AI
Robust ETL pipelines using Apache Airflow, Spark, and Kafka. Ensure your data is clean, accessible, and ready for machine learning workflows.
Featured Projects
GPU-Optimized Model Deployment for Healthcare
Deployed a real-time patient monitoring system using GPU-powered infrastructure. Reduced latency by 40% and achieved 24/7 availability with Kubernetes orchestration.
Key Achievements:
- 40% reduction in inference latency
- 99.99% uptime achieved
- Scalable to handle 10,000+ concurrent patients
Hybrid Cloud MLOps for Retail
Designed a scalable MLflow tracking system across on-premise and cloud environments. Enabled seamless CI/CD with 99.9% uptime for model training and deployment.
Technical Details:
- 20-node GPU cluster for distributed training
- 30% reduction in compute costs
- Automated A/B testing pipeline for continuous improvement
Latest Insights
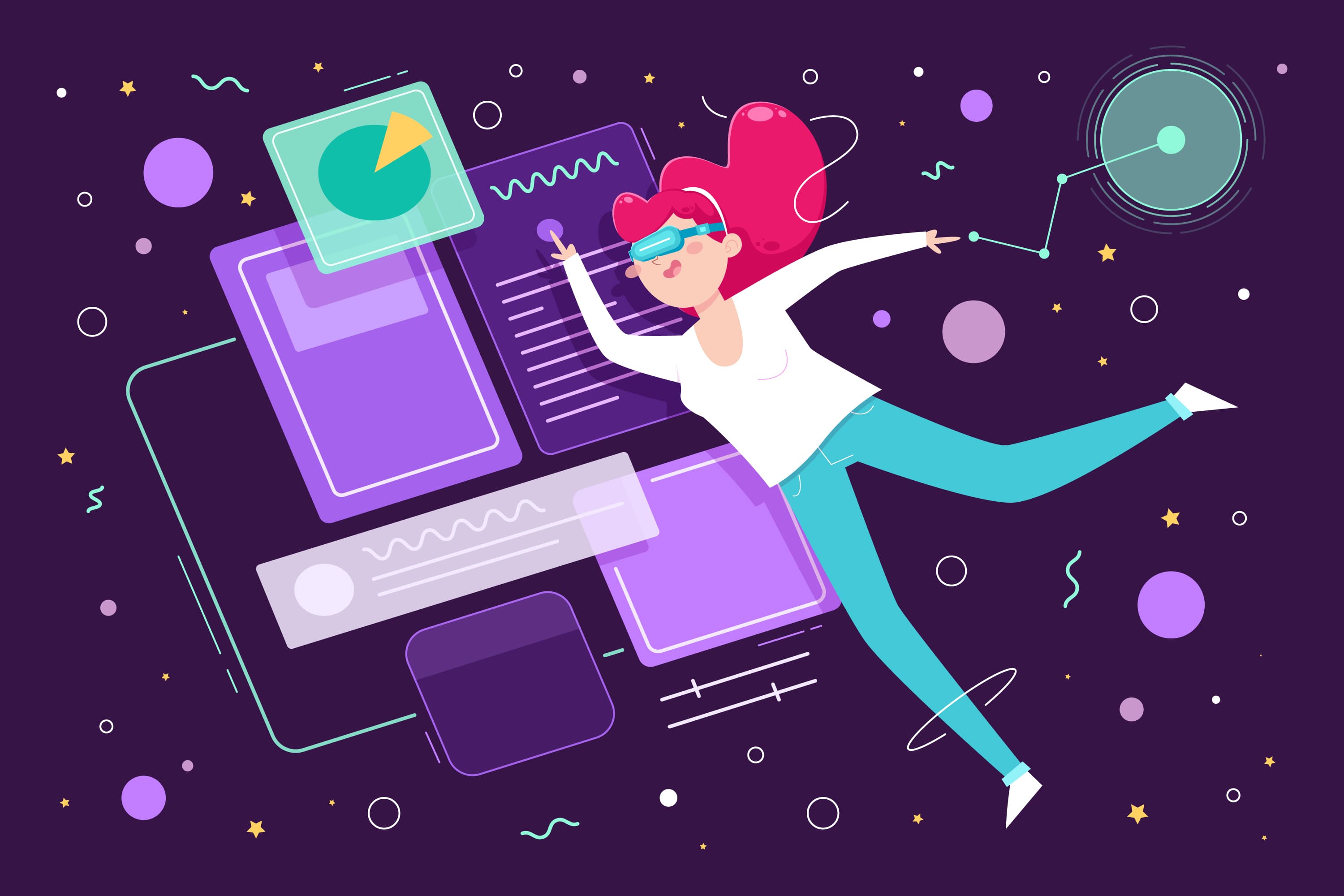
Top 5 MLOps Tools You Should Be Using in 2024
Discover the most powerful and efficient MLOps tools that are shaping the industry this year.
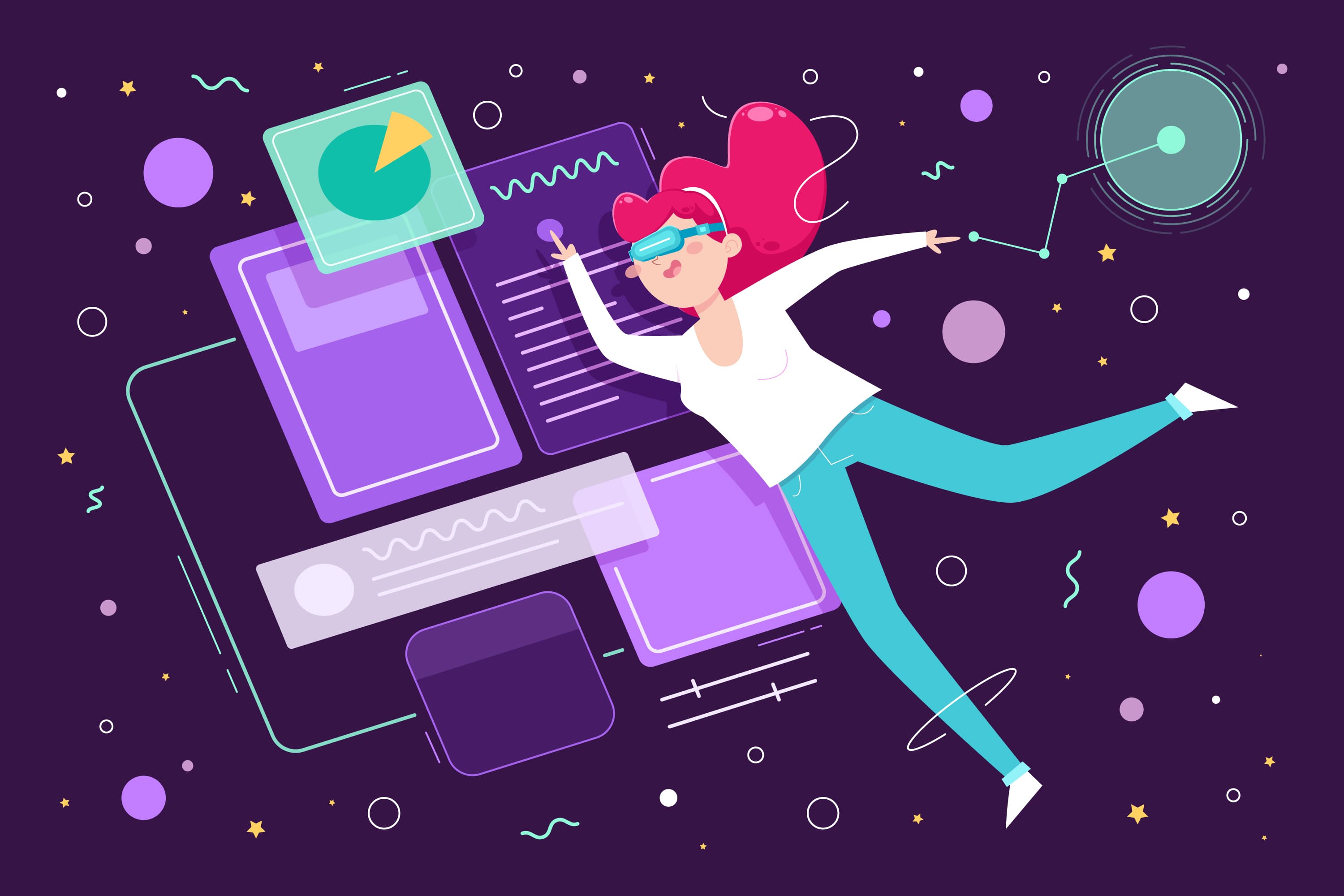
GPU Workload Optimization: Best Practices
Learn how to maximize the performance of your GPU clusters for AI training and inference.
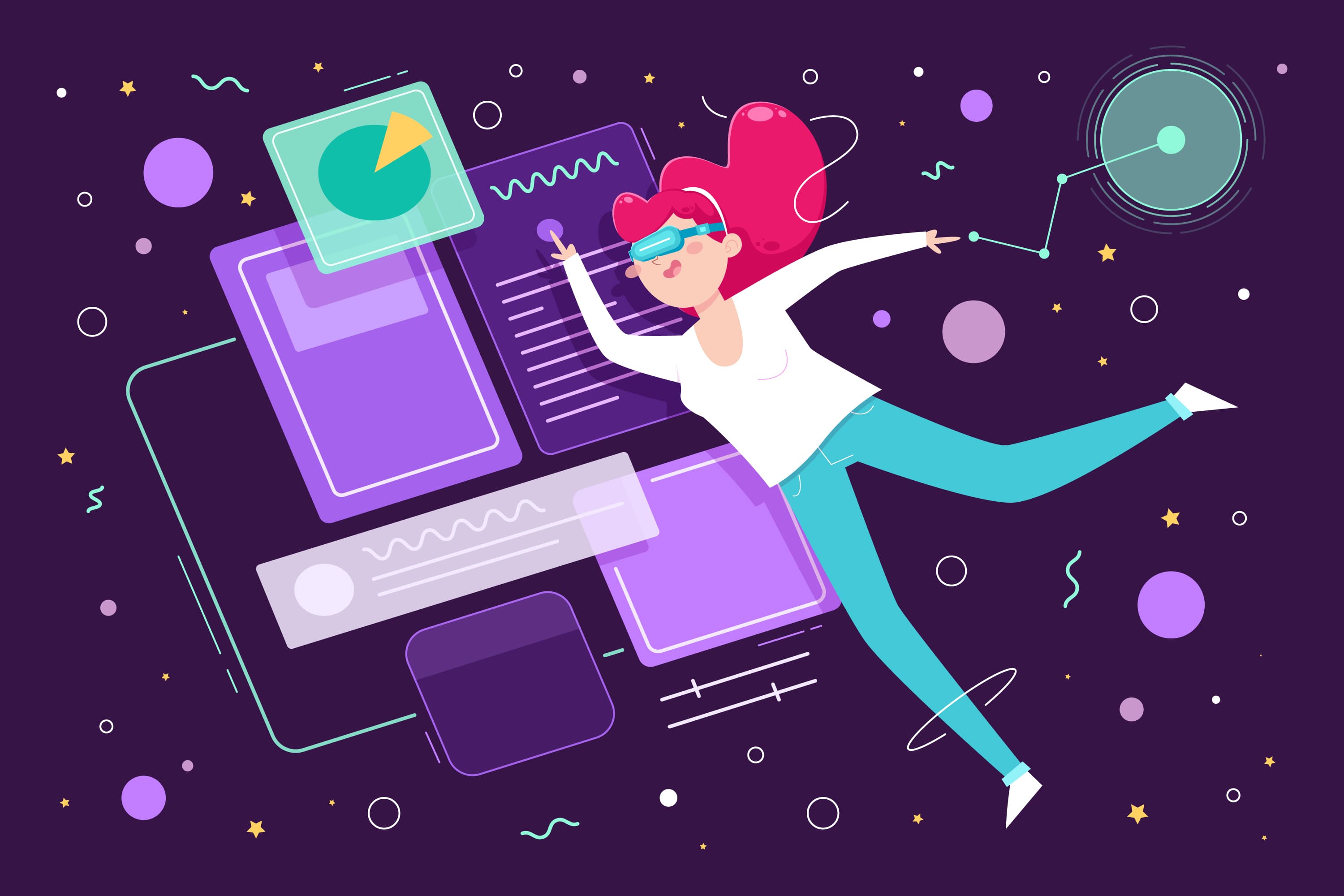
Scaling Machine Learning with Kubernetes
A comprehensive guide to leveraging Kubernetes for scalable and efficient ML workflows.
Downloadable Resources
The Future of MLOps in Cloud-Native Environments
Explore the trends and technologies shaping the future of MLOps in this comprehensive whitepaper.
MLOps Pipeline Workflow Template
Get started quickly with our customizable MLOps pipeline workflow template.
Case Study: AI in Healthcare
Learn how we implemented a scalable MLOps solution for a leading healthcare provider.